HomeSense is a methodological research project focused on the use of digital sensors in social research. One of the key issues is finding meaningful information in sensor-generated data, which was the focus of a paper presented by Jie Jiang at the International Conference on Future Networks and Distributed Systems (ICFNDS’17) in Cambridge in July this year.
The paper now published in the conference proceedings, covers data analysis from the early stages of the HomeSense field trial. It reports on applying machine learning methods to interpret sensor-generated data, and discusses a method for identifying features of various types of activity and evaluating the agreement between sensor-generated data and self-reported data from time-use diaries.
Since one of the implications of using sensors for social research is that, in due course, activities could be recognised automatically, this study also proposes a method for modelling a range of activities recorded by sensors.
The same approach has been continued by the team in an extended version of the study with data from more households, but the publication of the conference paper in the Proceedings of ICFNDS’17 provided an opportunity for some of its authors – Jie Jiang, Riccardo Pozza, Kristrún Gunnarsdóttir – to sit down and discuss the implications of this work, and where it sits within the project as a whole.
The extended study, “Using Sensors to Study Home Activities“, is now published in the Journal of Sensors and Actuator Networks 6(4): 32.
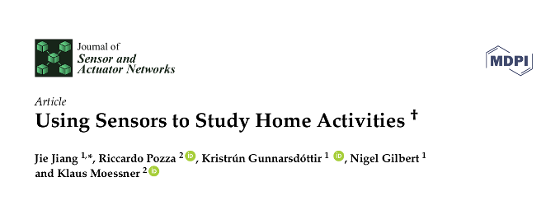
Establishing a framework to evaluate agreement between two different sources of data
The extended paper reports on a pilot of three households using the same data-analytic methods, that, according to Jie Jiang, provides, “a framework to evaluate agreement between different sources of data that recognises activities of people’s daily lives.”
While activity studies generally ask participants to consider their actions in talk or by writing them down in retrospect, to mitigate the likely inconsistencies in such records, researchers can apply additional methods to pinpoint what people are ‘actually‘ doing and when, known as ‘ground truth’.
“Typically”, added Jie, “studies seek to establish ‘ground truth’ by use of cameras or they give people a predefined list of tasks to do at a particular time.”
In our paper however, rather than considering the exact details of the timings of activities, this paper simply considers the methodological question of quantifying the degree of agreement between the sensor data and corresponding participant records written in a time/use diary, and it formulates a framework for evaluating to what extent they agree.
In other words, the purpose was not to find the ‘truth’ of what happened and when, but merely address a way to measure the differences in what the two methods deliver and how to interpret the results.
Kristrún added, “In this work, using time-use diaries and sensor-generated data, you can ease on the expectation of ground truth to create a framework that simply explores agreements.”
“That’s unique.”
”New methods need to be introduced gradually“, Jie said, “so understanding similarities and differences in using sensor generated data as opposed to traditional methods is an important challenge for HomeSense.”
“As we don’t yet know how useful digital sensors are to social researchers or how to use this method in social settings, we have to evaluate in both the traditional way and with the new method, and see how they agree with each other. This can give some confidence of how well sensor-generated data can be used for understanding such things as daily activities.”
Advantages of sensor-generated data for social science
“Actually, there are many methods for recognising activities from sensor generated data”, added Jie.
And anyway, time-use diary data is not likely to be entirely accurate because it records people recollections after the event, every 10 minutes or so, whereas sensor-generated data is recorded automatically every 3 seconds for each data point.
“There’s also the advantage in practical terms of overcoming the privacy issues of using camera data”, added Riccardo Pozza.
“Not many people would want to have cameras monitoring all their activities in a private household at all times because they might be doing things they don’t want you to see.”
Data model based on mean-shift clustering and change-point analysis
Going deeper into the model, Riccardo said,“We applied machine learning techniques for pattern recognition to understand patterns from the data and assign these to activities of daily living of the subject at home, e.g. eating, cooking, playing with a computer, etc.”
Instead of evaluating the model against some ‘ground truth’ the researchers decided on an approach training a model to derive meaning from the data.
”We extracted features from raw data via mean-shift clustering as a way of taking the sensor-generated data and ‘clustering’ it around its average.”
“Clustering is an area of unsupervised machine learning, based on dividing data into groups without requiring knowledge about what each group represents.”
“Mean-shift clustering proved very effective for grouping sensor values around their mean values, to eliminate spurious fluctuations in the data and more meaningfully describe the features of the data.”
“For example, a sound level sensor would record changes in measured decibels, but only an average value over a time window is needed to recognise presence or absence of noise to interpret presence in a room, such as when someone is cooking a meal in a kitchen.”
“We could produce a model recognising what the data revealed, using machine learning, and train the model to be more reliable by presenting it with loads of data over time.”
New combination of methods for social research
“Actually“, said Jie, ”there are many similar methods we could use to recognise activities from sensor-generated data”.
”Also, both human subjects and learning models might misreport activities and people simply might not want to report more sensitive activities.”
“And the question about which method is more accurate is a very difficult question to answer… but we only need to know to what extent we can use sensor-generated data.”
Methodological implications
The feedback received by Jie at ICFNDS’17, from an audience of mainly engineers, naturally homed in on the technology rather than what it could be used for.
“They asked about the technical methodology rather than social science, such as the Hidden Markov Models, and about the types of sensors used in the study”.
Looking ahead to further possibilities of the field trial and the data the project can generate, Jie continued, “we’ve now started to include more sensors including Bluetooth connectivity… and we’re still recruiting more households to further explore the method.” Rounding up, Kristrún characterised the implications of this work in the whole as purely methodological… “which for the scholarly community is significant; it’s an end in itself for pushing methodological developments forward.”
Using Sensors to Study Home Activities. Journal of Sensors and Actuator Networks 6(4): 32.